In today’s fast-paced digital era, the integration of machine learning in healthcare is rapidly transforming how medical professionals diagnose, treat, and monitor patients. This revolutionary technology is paving the way for new levels of efficiency and precision in the medical field. In this comprehensive article, we will explore machine learning in healthcare from every angle—its applications, benefits, challenges, and future prospects. Whether you are a healthcare professional, a technology enthusiast, or simply curious about the latest in medical innovation, this article is designed to provide you with valuable insights into how machine learning in healthcare is reshaping patient care and driving monumental change in the industry.
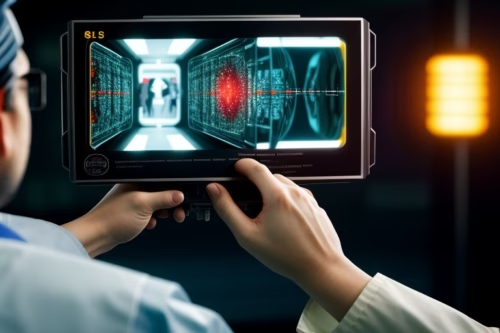
Introduction to Machine Learning in Healthcare
At its core, machine learning in healthcare involves the use of algorithms and statistical models that enable computers to perform tasks without explicit instructions. By analyzing vast amounts of medical data, these algorithms can detect patterns and predict health outcomes, leading to early diagnosis, personalized treatment plans, and improved patient management. The transformative power of machine learning in healthcare is not just limited to diagnostics but extends to various aspects of patient care including imaging, genomics, and operational efficiencies within healthcare facilities.
This integration of technology into medicine is nothing short of a revolution. With advancements in data analytics and computing power, machine learning in healthcare is set to redefine what is possible in medicine. For additional information on the evolution of this technology, check out the Harvard Business Review article on ML breakthroughs.
Why Machine Learning in Healthcare is a Game-Changer
The true strength of machine learning in healthcare lies in its ability to analyze extensive datasets that far exceed human capacity. By processing thousands of medical records, research studies, and clinical trial results, these algorithms can identify trends and correlations that often go unnoticed. Here are some of the primary benefits:
-
Enhanced Diagnostic Accuracy: Machine learning in healthcare systems can help in identifying diseases at an early stage, sometimes even before symptoms appear. For instance, sophisticated image recognition algorithms can analyze X-rays or MRI scans to spot anomalies with remarkable precision.
-
Personalized Treatment: With the ability to assess individual patient data, machine learning in healthcare tailors treatment plans based on the unique genetic makeup and medical history of each patient. This level of customization improves treatment outcomes and minimizes adverse reactions.
-
Operational Efficiency: Hospitals and clinics are leveraging machine learning in healthcare to streamline administrative tasks, optimize resource allocation, and reduce operational costs. This not only enhances the patient experience but also allows healthcare providers to focus more on patient care rather than paperwork.
-
Predictive Analytics: By forecasting potential health issues before they become critical, machine learning in healthcare enables proactive care. This predictive capability can lead to significant improvements in patient management and long-term health outcomes.
For a deeper dive into predictive analytics and technology in medicine, you can refer to research shared on NIH’s official website.
Transformative Applications of Machine Learning in Healthcare
1. Medical Imaging and Diagnostics
Medical imaging is one of the most notable applications of machine learning in healthcare. Advanced algorithms are used to interpret X-rays, CT scans, and MRIs with high accuracy. These algorithms not only assist radiologists in detecting abnormalities but also reduce human error. With features like automated image recognition and anomaly detection, these tools are especially valuable in diagnosing cancers, neurological disorders, and cardiovascular diseases.
Recent studies have shown that machine learning in healthcare can outperform traditional methods in certain diagnostic tasks. The ability to analyze thousands of images in seconds means that diagnostic procedures become faster and more reliable. For further reading on advancements in imaging, explore the latest insights on Radiology Today.
2. Genomics and Precision Medicine
The field of genomics stands at the frontier of personalized medicine. Machine learning in healthcare plays a crucial role in decoding the genetic information that underpins many diseases. By analyzing genomic data, algorithms can identify mutations and genetic markers that influence treatment responses. This helps in developing targeted therapies that are tailored to individual genetic profiles.
This form of precision medicine reduces the trial-and-error approach often seen in traditional treatments and enhances the efficacy of therapies by selecting the right medication for the right patient. Interested readers can discover more about this topic through the CDC’s genomic research initiatives.
3. Virtual Health Assistants and Chatbots
The integration of machine learning in healthcare has led to the emergence of virtual health assistants and chatbots that support patient care around the clock. These tools leverage natural language processing (NLP) to understand patient queries, provide medical advice, and even schedule appointments. They serve as the first point of contact in many healthcare systems, easing the burden on clinical staff and improving accessibility to care.
The adoption of these virtual assistants has seen significant growth, particularly during times when in-person consultations are limited. They also facilitate chronic disease management by offering reminders for medication and tracking patient symptoms over time. For more on virtual healthcare innovations, check out insights available on MobiHealthNews.
4. Drug Discovery and Development
Developing new drugs is a time-consuming and costly endeavor. Machine learning in healthcare has dramatically accelerated this process by predicting the efficacy and safety of drug candidates. Algorithms can quickly screen vast libraries of chemical compounds to identify potential drugs, thereby reducing the time required for preclinical testing.
Moreover, predictive models help in understanding how different compounds interact within the human body, enabling researchers to foresee side effects and optimize drug formulations even before they enter clinical trials. This revolution in drug discovery not only speeds up the process but also lowers research and development costs. To learn more about how technology is reshaping drug discovery, visit Forbes Life Sciences.
5. Hospital Operations and Workflow Optimization
Beyond clinical applications, machine learning in healthcare has a significant impact on hospital operations. From managing patient flow to streamlining supply chain logistics, these smart systems help healthcare institutions operate efficiently. Predictive analytics in hospital management can forecast patient admissions, reducing wait times and improving emergency response. This operational efficiency directly translates into better patient care and enhanced resource utilization.
Another key advantage is the potential for reducing administrative burdens through automation. Tasks such as billing, appointment scheduling, and patient data management are increasingly being automated, allowing healthcare professionals to focus on patient-centric care. More details on operational improvements can be found in articles on Healthcare IT News.
6. Remote Monitoring and Wearable Technology
The proliferation of wearable devices—ranging from smartwatches to advanced biosensors—has created a vast repository of real-time patient data. Machine learning in healthcare is essential in analyzing this data to monitor chronic conditions, detect early signs of illness, and provide real-time feedback. Continuous monitoring enables early interventions and personalized care strategies based on day-to-day changes in a patient’s health profile.
With the integration of remote monitoring, particularly during public health emergencies, healthcare providers can maintain constant oversight of vulnerable populations without physical interaction. For additional insights on wearable technology and remote health monitoring, explore TechCrunch’s coverage of digital health.
7. Enhancing Patient Engagement and Health Outcomes
Perhaps one of the most profound impacts of machine learning in healthcare is its ability to enhance patient engagement. Personalized health recommendations, automated follow-up reminders, and interactive health education programs are all driven by sophisticated algorithms. These tools empower patients by making them active participants in their health management. This engagement is key to improving long-term health outcomes and reducing the incidence of preventable diseases.
Innovative patient engagement platforms use machine learning to analyze patient feedback and adjust their services in real time. This results in a more responsive healthcare system that continuously adapts to the needs of its patients. To see how these innovations are making a difference, read more on Healthline’s patient engagement strategies.
Challenges Facing Machine Learning in Healthcare
Despite its many benefits, machine learning in healthcare is not without challenges. Several factors complicate its implementation and widespread adoption:
Data Quality and Privacy:
The success of machine learning in healthcare hinges on access to high-quality, well-labeled data. However, medical data is often fragmented, inconsistent, and subject to strict privacy regulations. Ensuring that data used in training algorithms is accurate, secure, and ethically sourced is paramount. Data privacy concerns also play a significant role—healthcare providers must balance the need for comprehensive data with rigorous patient confidentiality measures under regulations like HIPAA.
Interoperability Issues:
Healthcare systems are notorious for their complex, siloed IT environments. Integrating machine learning in healthcare into these disparate systems can be a daunting task. Seamless interoperability between different electronic health record (EHR) systems is crucial for the success of machine learning applications. Without proper integration, the full potential of these algorithms cannot be realized.
Algorithm Bias and Fairness:
Another critical concern is the risk of algorithmic bias. If the data used to train machine learning in healthcare models is not representative of the entire population, the resulting algorithms may inadvertently produce biased outcomes. This bias can lead to disparities in care, particularly for minority groups. Ongoing research and the development of ethical guidelines are essential to ensure that machine learning tools provide fair and unbiased healthcare solutions.
Regulatory and Ethical Considerations:
The integration of machine learning in healthcare brings forth complex regulatory and ethical challenges. Healthcare providers and tech companies must navigate a labyrinth of regulations governing the use of artificial intelligence in patient care. Ethical considerations such as patient consent, transparency, and accountability are central to the responsible use of these technologies. Policymakers and industry leaders are continuously working to develop frameworks that ensure the safe and equitable implementation of these systems. For a detailed discussion on ethical AI, see MIT Technology Review.
Successful Case Studies of Machine Learning in Healthcare
Real-Life Implementation in Radiology
One of the most compelling success stories of machine learning in healthcare comes from radiology departments across the globe. Hospitals that have integrated AI-driven diagnostic tools report significant reductions in diagnostic errors and faster turnaround times for imaging results. In some cases, radiologists are now able to detect intricate details in scans that were previously missed. This success not only enhances the quality of care but also reduces the burden on radiology staff, allowing them to devote more time to complex cases.
Innovations in Oncology
In oncology, machine learning in healthcare is being used to predict patient responses to cancer treatments by analyzing historical patient data. This predictive capability has been a game-changer in designing personalized treatment plans. By matching the right therapies to the right patients, oncologists can improve survival rates and reduce adverse effects. Innovative cancer centers that utilize these systems have reported improvements in treatment efficacy, serving as excellent examples of technology-driven patient care.
Transforming Chronic Disease Management
For patients with chronic conditions such as diabetes and heart disease, continuous monitoring and timely intervention are critical. Machine learning in healthcare applications that monitor patient data in real time have enabled healthcare providers to predict complications and intervene early. This proactive approach has significantly reduced hospital readmission rates and improved overall patient health. Many hospitals are now partnering with tech companies to develop integrated chronic disease management platforms that rely heavily on machine learning models.
Revolutionizing Drug Discovery
Pharmaceutical companies are increasingly embracing machine learning in healthcare to revolutionize the drug discovery process. By using sophisticated algorithms to analyze vast chemical databases and predict the efficacy of new compounds, researchers are now able to develop innovative drugs more quickly than ever before. This leap in efficiency has not only shortened the time to market for new treatments but has also paved the way for more targeted therapies in areas previously considered challenging. Detailed insights into drug discovery enhancements can be further explored on PharmaTimes.
Future Trends in Machine Learning in Healthcare
Looking ahead, the potential for machine learning in healthcare is vast and largely untapped. As computational power increases and datasets become richer and more diverse, we can expect to see a host of new applications emerging in the near future.
Integration with the Internet of Medical Things (IoMT):
The IoMT is set to revolutionize patient care by connecting an array of smart devices, wearables, and sensors to create a comprehensive ecosystem of patient data. Machine learning in healthcare will play a pivotal role in analyzing this continuous influx of data and providing actionable insights to healthcare providers.
Advancements in Natural Language Processing:
The future of machine learning in healthcare will also be shaped by significant improvements in natural language processing (NLP). These advancements will enable the development of systems that can accurately interpret and analyze unstructured clinical notes, patient records, and even real-time conversations between patients and clinicians. This will streamline documentation, reduce administrative burdens, and enhance clinical decision-making.
Enhanced Cybersecurity Measures:
As healthcare providers embrace machine learning in healthcare, the need to secure medical data becomes even more critical. Future developments will likely focus on integrating advanced cybersecurity protocols directly into machine learning systems, ensuring that sensitive patient data remains protected from breaches and cyberattacks.
Collaboration Between Industry and Academia:
To fully harness the transformative potential of machine learning in healthcare, collaboration between industry stakeholders and academic institutions is essential. Joint research initiatives and shared data platforms will accelerate innovation and help in developing robust, ethical, and effective machine learning solutions tailored for healthcare. For further insights into academic collaborations and innovations, explore research updates on ScienceDirect.
Best Practices for Implementing Machine Learning in Healthcare
For healthcare providers who wish to harness the power of machine learning in healthcare, there are several best practices to keep in mind:
-
Invest in High-Quality Data:
The foundation of any successful machine learning system is the quality of the data it is built upon. Healthcare providers should invest in robust data collection and maintenance strategies to ensure that the datasets used in training are comprehensive and reliable. -
Ensure Interoperability:
It is crucial to design systems that can seamlessly integrate with existing healthcare IT infrastructures. This involves working closely with vendors and technology experts to develop solutions that are compatible with multiple electronic health record (EHR) systems. -
Prioritize Data Security and Privacy:
With strict regulatory landscapes such as HIPAA, safeguarding patient data is non-negotiable. Implementing end-to-end encryption, access controls, and regular security audits are essential measures for protecting sensitive information. -
Adopt a Collaborative Approach:
The successful integration of machine learning in healthcare requires collaboration between clinicians, data scientists, and IT professionals. Regular training sessions and cross-disciplinary teams can help bridge the gap between technology and medicine. -
Stay Updated with Regulatory Guidelines:
The field of healthcare technology is rapidly evolving, and so are the regulations that govern it. Healthcare providers must stay informed about the latest guidelines and best practices to ensure compliance and ethical usage of data. -
Encourage Patient Engagement:
Engaging patients in their care journey can lead to better health outcomes. Utilizing patient-facing technologies, such as mobile apps and virtual health assistants, powered by machine learning in healthcare can improve patient satisfaction and treatment adherence.
Addressing the Ethical and Regulatory Landscape
While the benefits of machine learning in healthcare are immense, it is equally important to tackle the ethical and regulatory challenges head-on. Transparent data usage policies, clear consent protocols, and continuous monitoring for bias are essential to ensure that these innovative tools serve all segments of the population fairly. Healthcare institutions are increasingly adopting ethical guidelines and protocols to govern the use of machine learning tools, ensuring that patient trust is maintained even as technology evolves.
By actively engaging with regulatory bodies and ethical boards, tech companies and healthcare providers can build robust systems that are not only innovative but also trustworthy. This open approach is key to fostering long-term success in the adoption of machine learning in healthcare technologies. For further reading on ethical guidelines and regulatory challenges, see the reports available on World Health Organization.
Conclusion
The evolution of machine learning in healthcare marks a pivotal moment in the transformation of patient care. With the promise of enhanced diagnostics, personalized treatment, streamlined hospital operations, and revolutionary drug discovery, it is clear that this technology is not a passing trend but a foundational pillar for the future of medicine. As we continue to witness the rapid advancement of this field, the integration of sophisticated algorithms into everyday healthcare practices is set to drive significant improvements in patient outcomes while also reducing operational inefficiencies.
This comprehensive exploration into the realm of machine learning in healthcare demonstrates that embracing technology is not just about innovation—it is about saving lives, improving care quality, and building a smarter, more responsive healthcare system. The future beckons with opportunities to further integrate artificial intelligence into healthcare, ensuring that medicine becomes more predictive, personalized, and precise.
By implementing the best practices discussed, addressing ethical challenges head-on, and fostering collaboration between industry and academia, healthcare providers can unlock the full potential of machine learning in healthcare. The transformative nature of this technology is already evident in areas such as medical imaging, genomics, remote monitoring, and patient engagement. As these advancements become more widespread, the entire healthcare ecosystem will benefit—from doctors and researchers to patients and their families.
Embracing machine learning in healthcare is not without its challenges, but the potential benefits far outweigh the obstacles. With continuous improvements in data quality, regulatory compliance, and ethical frameworks, the road ahead is filled with promise. The future of healthcare is intertwined with the advances in artificial intelligence, and those who invest in these technologies today will lead the charge in shaping a healthier tomorrow.
For ongoing updates and further insights into the world of technology-driven healthcare, consider following reputable sources such as Nature Medicine and The Lancet Digital Health. These platforms provide timely information on breakthroughs, clinical trials, and the real-world impact of machine learning in healthcare.
In summary, as we embrace the digital revolution, machine learning in healthcare stands at the forefront of modern medicine. Its ability to revolutionize diagnostics, empower personalized treatments, and streamline hospital operations makes it an indispensable asset in our continuous pursuit of excellence in patient care. Now is the time for healthcare providers, researchers, and technologists to come together and harness the transformative power of machine learning in healthcare for a brighter, healthier future.
This article has explored seven transformative ways machine learning in healthcare is reshaping patient care. From enhancing diagnostic accuracy in medical imaging and enabling personalized treatment through genomics, to optimizing hospital operations and spearheading drug discovery, the potential of this technology knows no bounds. With virtual health assistants and remote monitoring devices becoming increasingly prevalent, the promise of a more efficient, patient-focused, and technologically advanced healthcare system is closer than ever.
The impact of machine learning in healthcare extends beyond clinical applications. As digital health technologies continue to evolve, the integration of artificial intelligence into everyday practice is set to redefine the roles of healthcare providers, empower patients with real-time health insights, and ultimately, drive down costs while improving quality of care. The future is bright, and the revolution is already underway.
To conclude, the journey of adopting machine learning in healthcare is paved with both opportunities and challenges. Embracing these advancements requires a careful balancing act—investing in high-quality data, ensuring robust cybersecurity, and maintaining a relentless commitment to ethical standards. As healthcare ecosystems worldwide navigate this complex landscape, the ultimate goal remains clear: to improve patient outcomes, enhance the quality of care, and build a sustainable future for global health.
By staying informed and actively participating in this technological revolution, we can ensure that machine learning in healthcare achieves its full potential as a transformative force in medicine. Let this comprehensive guide be a stepping stone towards a future where innovative technology and compassionate care come together for the benefit of all.
Embrace the change, and join the movement. The revolution in machine learning in healthcare is here—transforming lives, empowering clinicians, and paving the way for unprecedented advances in medical science. Stay ahead of the curve by continuing to explore, innovate, and implement the latest in healthcare technology. Your journey towards a smarter, more efficient healthcare system starts now.
For further reading and additional resources, check out these insightful external articles:
By harnessing the power of machine learning in healthcare, we are not just improving systems—we are improving lives. Join the revolution, and witness firsthand the transformative impact of this cutting-edge technology on the future of patient care.